class: inverse, center, middle background-image: url(http://cc.oulu.fi/~scoats/oululogoRedTransparent.png); background-repeat: no-repeat; background-size: 80px 57px; background-position:right top; exclude: true --- class: title-slide, center <br> ## Regional Variation in Speech Rate in American English from YouTube Videos ### Steven Coats <br><br><br> English Philology, University of Oulu, Finland<br> <a href="mailto:steven.coats@oulu.fi">steven.coats@oulu.fi</a><br> RDHum Conference, Oulu<br> August 14th, 2019<br> --- layout: true <div class="my-header"><img border="0" alt="W3Schools" src="http://cc.oulu.fi/~scoats/oululogonewEng.png" width="80" height="80"></div> <div class="my-footer"><span>Steven Coats         Regional Variation in Speech Rate in American English from YouTube Videos | RDHum, Oulu</span></div> --- <div class="my-header"><img border="0" alt="W3Schools" src="http://cc.oulu.fi/~scoats/oululogonewEng.png" width="80" height="80"></div> <div class="my-footer"><span>Steven Coats         Regional Variation in Speech Rate in American English from YouTube Videos | RDHum, Oulu</span></div> ## Outline 1. Corpus sociophonetics, speaking and articulation rate, previous work, research questions 2. Data collection from YouTube, transcript files, corpus creation 3. Calculation of articulation rate 4. Spatial autocorrelation: Getis-Ord G<span class='supsub'><sup>*</sup><sub>i</sub></span> for regional analysis, urban-rural diffences 5. Caveats, summary, future outlook -- - New method for the calculation of articulation rate from automatic speech-to-text transcripts - Investigation of articulation rate vs. location, articulation rate vs. locality population - Mapping with local autocorrelation statistics .footnote[Slides for the presentation are on my homepage at https://cc.oulu.fi/~scoats] --- <div class="my-header"><img border="0" alt="W3Schools" src="http://cc.oulu.fi/~scoats/NewLogoRussianPNG1.png" width="80" height="80"></div> <div class="my-footer"><span>Steven Coats         Regional Variation in Speech Rate in American English from YouTube Videos | RDHum, Oulu</span></div> ### Corpus sociophonetics Prosodic features increasingly being considered as bearing indexicality in the same manner as (e.g.) lexical or grammatical variables .small[(Ray & Zahn, 1999; Kendall, 2013)] -- Attitudes about regional or urban-rural differences in speech temporality are common in the U.S. .small[(Preston, 1989, 1999; Roach, 1998)] Faster speech can be associated with - Competence, intelligence, and expertise .small[(Smith et al., 1975; Street & Brady, 1982; Thakerar & Giles, 1981)] - Persuasiveness .small[(Apple et al., 1979; Giles & Powesland, 1975, Miller et al., 1976)] - Attractiveness .small[(Street et al., 1983)]</br> compared to slower speech --- ### Example video (slow talker) <iframe width="560" height="315" src="https://www.youtube.com/embed/mUZPl-Y7eWI?start=4&end=17&?rel=0&&showinfo=0" frameborder="0" allow="accelerometer; autoplay; encrypted-media; gyroscope; picture-in-picture" allowfullscreen></iframe> --- ### Example video (fast talker) <iframe width="560" height="315" src="https://www.youtube.com/embed/BBSA-9GTffg?start=2&end=15&t=1rel=0&&showinfo=0" frameborder="0" allow="accelerometer; autoplay; encrypted-media; gyroscope; picture-in-picture" allowfullscreen></iframe> --- ### Speaking rate and articulation rate *Speaking rate*: Sum of units of speech (e.g. phones, syllables, or words) divided by total utterance time *Articulation rate*: Sum of units of speech divided by total utterance time, **omitting pauses between segments of unbroken speech** - Pause duration has been shown to vary .small[(Goldman-Eisler, 1961)], also according to demographic and regional parameters .small[(Clopper & Smiljanic, 2011, 2015)] - In this study **articulation rate**, measured in σ/sec., is compared --- ### Factors that can affect articulation rate - Type of speech: Reading, monologue, conversation - Conversation: Interlocutor familiarity, topic under discussion .small[(Yuan, Liberman & Cieri, 2006)] - Utterance-internal considerations .small[(Byrd & Saltzman, 1998; Yuan, Liberman & Cieri, 2006; Oller, 1973)] - Anatomical, physiological, or neurological parameters .small[(Tsao & Weismer, 1997; Tsao, Weismer & Iqbal, 2006)] - Demographic, social, or **regional** identity .small[(Byrd, 1992, 1994; Jacewicz et al., 2009, 2010; Kendall, 2014)] --- ### Previous work on regional variation in speech rate in the US .small[630 speakers reading 2 short sentences. Speaking rate measured. Results: **North > South** .small[(Byrd, 1992, 1994).] Problems: Locations of speakers not noted, only regional affiliation, non-naturalistic speech, small sample size. 92 speakers from Wisconsin and North Carolina reading test sentences and producing spontaneous speech. Articulation rate measured. Results: **Wisconsin > N. Carolina**. .small[(Jacewicz et al., 2009, 2010)]. Problems: Small sample size, regional inferences based on two locations. 42 young adults from Tennessee, New York State, and Nevada read a 266-word passage. Articulation rate measured. **Nevada > New York > Tennessee** .small[(Kendall, 2013)]. Problems: Small sample size, non-naturalistic speech, regional inferences based on three locations. 159 speakers, 30,136 utterances from sociolinguistic interviews. Articulation rate measured. **Texas, Ohio > North Carolina, Washington D.C.** .small[(Kendall, 2013)]. Problems: Regional inferences based on four states. 60 undergraduate students. Articulation rate measured. **New England > Midwest > South** .small[(Clopper & Pisoni, 2006; Clopper & Smiljanic, 2011, 2015)]. Problems: Regions based on small samples.] Trend evident, but - Low granularity - Non-naturalistic data - Small samples --- ### Research questions - Can we confirm the previous inferences on the basis of much larger data sets? - Are there differences in the temporality of urban/rural speech in the United States? --- ### Data source - Naturalistic language data on YouTube - Beginning in 2009, English-language videos accompanied by automatically generated speech-to-text captions .small[(Google, 2009)] with individual word timestamps - Recent advances in neural-network-based speech-to-text transcription increase transcript accuracy .small[(Chiu et al., 2018)] - High audio fidelity, standard language = accurate transcript = accurate word timings - Word timings can be leveraged to calculate articulation rate --- ### Example video <iframe width="560" height="315" src="https://www.youtube.com/embed/WY9RPeXA3pw?start=6&end=20&rel=0&&showinfo=0&cc_load_policy=1&cc_lang_pref=en" frameborder="0" allow="accelerometer; autoplay; encrypted-media; gyroscope; picture-in-picture" allowfullscreen></iframe> --- ### .vtt file 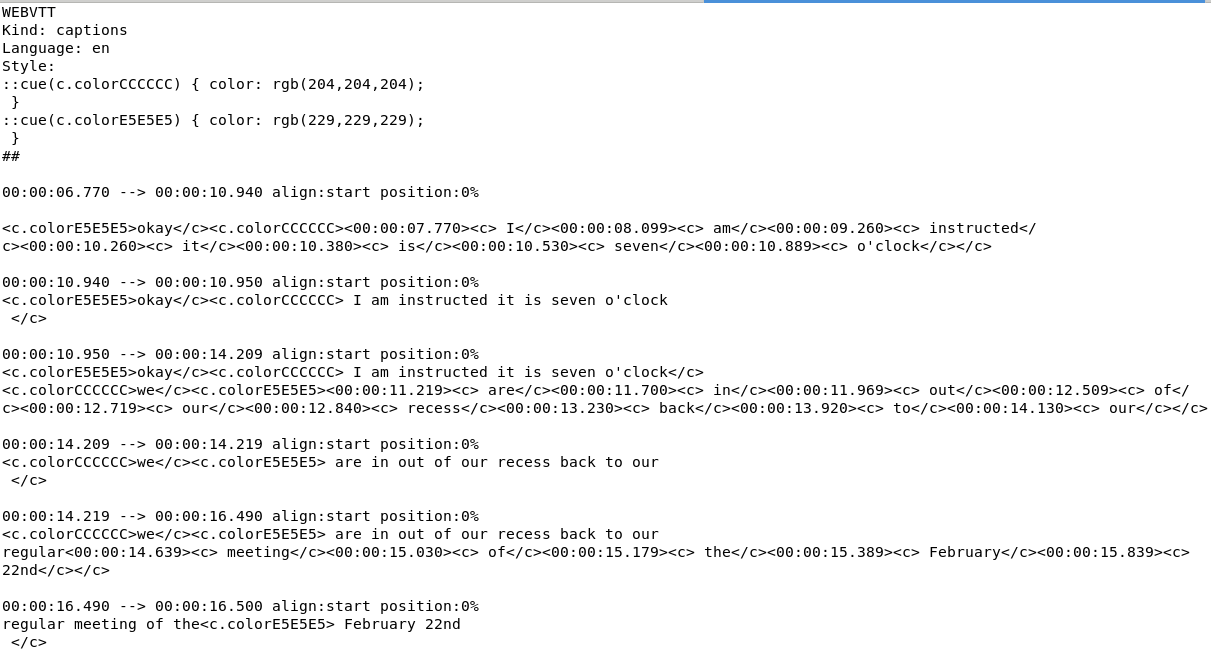 --- ### Focus on US local government/civic organization meetings Public meetings of elected representatives at town/city/county/state level: advantages in terms of representativeness and comparability - Speaker place of residence (cf. videos collected based on place-name search alone) - Topical contents comparable - Communicative contexts comparable - Audio quality often high --- ### Data collection and corpus Script to search YouTube API for channels: - Substrings `county of`, `city of`, `municipal`, `town meeting`, `city council`, `county supervisors`, `board of supervisors`, `government`, and `official government` + names/abbreviations 50 U.S. states or names of the 312 municipalities and 100 counties by population in the United States + corresponding state names/abbreviation - E.g. `county of Alabama`, `city council CA`, `official government Chicago, Illinois`, `official government Los Angeles County, California` - 1,680 channel matches, remove false positives and duplicates → 579 channels - Download all captions files (53,743) in .vtt format - Script to extract text and timings and assign exact latitude-longitude coordinates - Filtering: Remove channels with aggregate transcripts < 1,000 words, geographically delimit to 48 contiguous states - 49,345 videos; 28,166.77 hours of video; 235,824,795 words .small[(Coats, 2019)] --- ### Calculation of articulation rate - Words and their timing metadata are arranged sequentially in a captions block - Timings within a block do not overlap - If speech is continuous, approximate word durations (and articulation rate) can be calculated by subtracting a word's start time from that of the following word. --   BUT: the annotation does not explicitly indicate word ending, so silences within utterances or between utterances by different speakers = loooooong words AND: Utterance-initial words and those after long pauses are assigned a length of one second --- ### Calculation of articulation rate 2 <iframe width="560" height="315" src="https://www.youtube.com/embed/cK3CXpoH0qg?start=1050&end=1075&?hl=en&cc_lang_pref=en&cc_load_policy=1" frameborder="0" allow="accelerometer; autoplay; encrypted-media; gyroscope; picture-in-picture" allowfullscreen></iframe> .large[`00:17:30.820 --> 00:17:50.680` `it's<00:17:31.820> a<00:17:31.940> 3:3<00:17:32.660> vote<00:17:49.000> because<00:17:50.000> it's<00:17:50.150> a<00:17:50.240> personnel`] --- ### Calculation of articulation rate 3 Word | Start Time | End Time | Duration ------------- | ------------- | ------------ | ------------ it's | 17:30.820 | 17:31.820 | 1.000 a | 17:31.820 | 17:31.940 | .120 3:3 | 17:31.940 | 17:32.660 | .720 vote | 17:32.660 | 17:49.000 | 16.340 because | 17:49.000 | 17:50.000 | 1.000 it's | 17:50.000 | 17:50.150 | .150 a | 17:50.150 | 17:50.240 | .090 personnel | 17:50.240 | 17:50.680 | .440 Articulation rate: .610 σ/sec. = Not accurate --- ### Calculation of articulation rate 4 Word | Start Time | End Time | Duration ------------- | -------------- | -------------- | ------------ **it's** |**17:30.820**|**17:31.820**|**1.000** a | 17:31.820 | 17:31.940 | .120 3:3 | 17:31.940 | 17:32.660 | .720 **vote** |**17:32.660**|**17:49.000**|**16.340** **because** |**17:49.000**|**17:50.000**|**1.000** it's | 17:50.000 | 17:50.150 | .150 a | 17:50.150 | 17:50.240 | .090 personnel | 17:50.240 | 17:50.680 | .440 Articulation rate: 5.26 σ/sec. = Reasonable --- ### Calculation of articulation rate 5 - Filter out word tokens with long durations (utterance-initial words and words spoken immediately before or following longer pauses) #### Intra-utterance Continuous Articulation Rate - Articulation rate, in syllables per second, for all word tokens in a captions file whose sequential duration is less than 1 second - Validation of method: Use Praat script *speechrate* .small[(De Jong & Wempe, 2009)] to calculate articulation rate directly from audio files for random sample of 20 videos from corpus, Pearson's `\(r = 0.83\)` --- exclude: true ### Validation of method - Count syllables in Praat using *speechrate* .small[(De Jong & Wempe, 2009)] 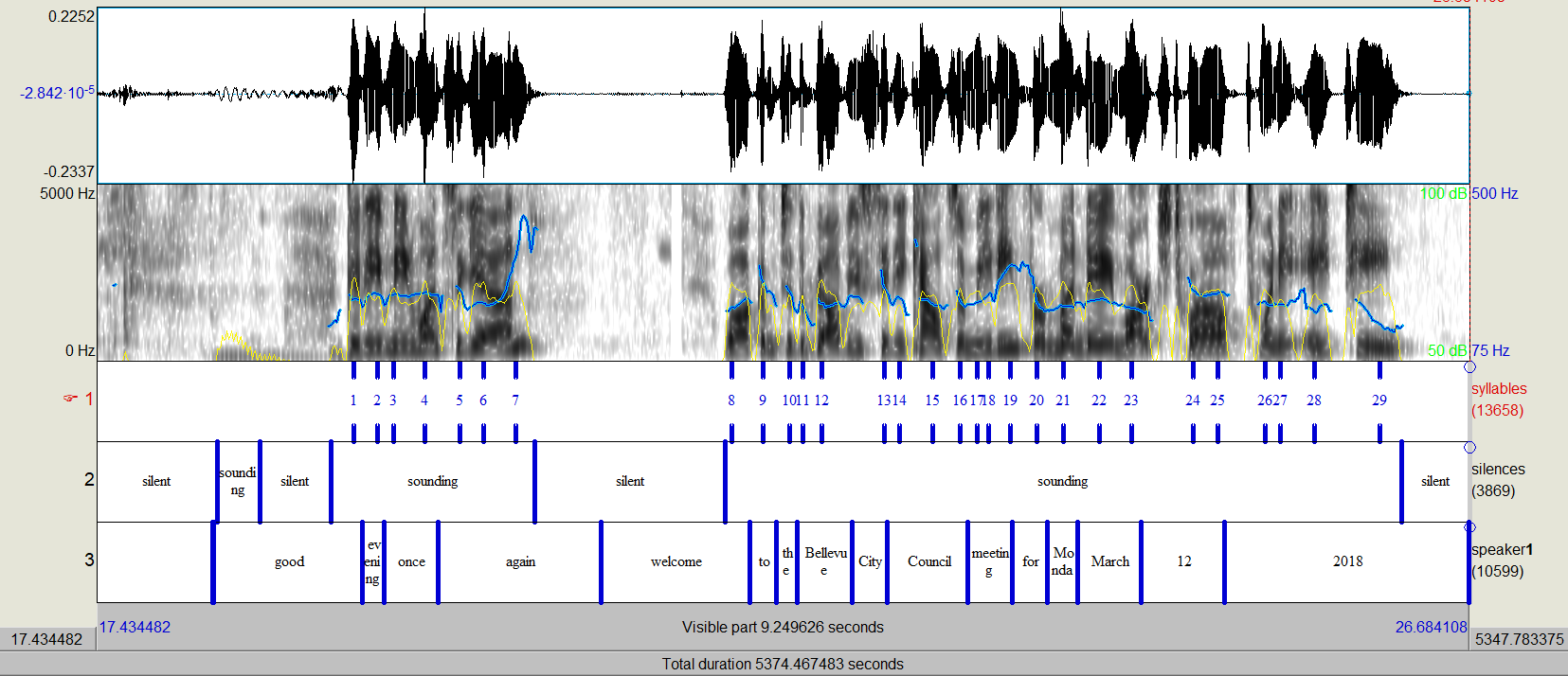 --- ### Regional analysis - For each channel location: Calculate the mean intra-utterance continuous articulation rate, based on all videos from that channel - Use the local spatial autocorrelation statistic Getis-Ord G<span class='supsub'><sup>*</sup><sub>i</sub></span> to infer large-scale patterns of difference or similarity - Map the variate into a Voronoi tesselation <img src="voro2.png" height = "350" /> --- ### <font>Getis-Ord G<span class='supsub'><sup>*</sup><sub>i</sub></span></font> <font size='3.5'>(Ord & Getis, 1992; Getis & Ord, 1995)</font> Local spatial autocorrelation statistic used in geography and recently in dialectology .small[(e.g. Grieve, 2016)] For each point in spatially distributed data: Positive value ➜ in a cluster of high values, negative value ➜ in a cluster of low values, zero ➜ not in a cluster -- `$$G_i^* = \frac{\sum_{j=1}^{n} w_{ij}x_j- \bar{x}\sum_{j=1}^{n} w_{ij}}{{\sqrt{\frac{\sum_{j=1}^{n} x_j^2} {n}-\bar{x}^2}}\sqrt{\frac{n\sum_{j=1}^{n} w_{ij}^2 -{(\sum_{j=1}^{n} w_{ij})^2}}{n-1}}}$$` .small[ `\(n\)` = number of locations, `\(x_{j}\)` = value of variable at location `\(j\)`, `\(w_{ij}\)` = value of spatial weights matrix for locations `\(i\)` and `\(j\)`, `\(\bar{x}\)` = mean of `\(x\)` at all locations ] Result is a standard deviate (significant at `\(p = 0.05\)` for `\(G_i^*\geq\pm1.645\)`) -- .small[ - **Spatial weights matrix** can be binary based on choropleth contiguity, nearest neighbors, or a cutoff distance, or continuous based on inverse distance or some other function] --- exclude: true ### Varying spatial weights matrices - Binary matrices based on choropleth contiguity, fewer nearest neighbors, or a small cutoff distance tend to reveal **more local patterns**, as do continuous matrices based on small inverse distances - Binary matrices based on more nearest neighbors or a larger cutoff distance tend to reveal **broader, supra-regional patterns**, as do continuous matrices based on large inverse distances --- <div class="my-header"><img border="0" alt="W3Schools" src="http://cc.oulu.fi/~scoats/NewLogoRussianPNG1.png" width="80" height="80"></div> <div class="my-footer"><span>Steven Coats         Regional Variation in Speech Rate in American English from YouTube Videos | RDHum, Oulu</span></div> ### Regional analysis .verysmall[https://stcoats.github.io/artic_rate_new.html] <div class="midcenter"> <iframe src="https://cc.oulu.fi/~scoats/artic_rate_rdhum.html" sandbox="allow-same-origin allow-scripts" width="960px" height="550px" scrolling="no" seamless="seamless" frameborder="0" align="top"> </iframe> .vsup[(Weights matrices: polygon continuity binary; 5, 10, 25, and 50 nearest-neighbor binary; and inverse distance with cutoffs of 200km and 100km)] </div> --- ### Regional findings - Spatial autocorrelation analysis suggests lower articulation rates in the South (Mississippi, Alabama, Tennessee) and higher rates in the Upper Midwest (Wisconsin, Minnesota, Dakotas), the Mountain West, and parts of Florida --- ### Urban-rural difference .small[ - Script to extract place names associated with the latitude-longitude coordinates for each channel, population estimates in data from the U.S. Census Bureau (U.S. Census Bureau, 2017) - Linear regression of articulation rate and log population] <img src="log_pop_art_rateAug_crop.png" height = "350" /> --- ### Caveats - Many different video genres represented in channels (not only meetings) - Meetings of local government not representative of speech in general? - Measure dependent on quality of transcript ( = quality of audio) - Large degree of variation within small regions (patterns only emerge using spatial autocorrelation statistic) --- ### Summary and outlook - Large corpus of automatic speech-to-text transcripts from YouTube channels of local governments - New method to calculate articulation rate from word timing information - Spatial autocorrelation/visualization shows that - Southerners speak slightly more slowly - People in cities speak slightly faster -- *** - Indexicality of articulation rate - Look in the transcripts for lexical items known to index regional identity (dialect words), regress frequencies with articulation rate - Pause frequency and duration analysis - Automatic identification of higher-quality (more accurate) transcripts - Automatic annotation of speaker variables? --- #Thank you! --- ### References .verysmall[ .hangingindent[ Apple, W., Streeter, L. A., & Krauss, R. M. 1979. Effects of pitch and speech rate on personal attributions. *Journal of Personality and Social Psychology* 37, 715–27. Byrd, D. 1992. Preliminary results on speaker-dependent variation in the TIMIT database. *Journal of the Acoustical Society of America* 92, 593–596. Byrd, D. 1994. Relations of sex and dialect to reduction. *Speech Communication* 15, 39–54. Byrd, D., & Saltzman, E. 1998. Intragestural dynamics of multiple phrasal boundaries. *Journal of Phonetics* 26, 173–199. Chiu, C.-C., Sainath, T., Wu, Y., Prabhavalkar, R., Nguyen, P., Chen, Z., Kannan, A., Weiss, R. J., Rao, K., Gonina, E., Jaitly, N., Li, B., Chorowski, J., & Bacchiani, M. 2018. State-of-the-art speech recognition with sequence-to-sequence models. [arXiv:1712.01769v6 [cs.CL]](https://arxiv.org/pdf/1712.01769.pdf). Clopper, C. G., & Pisoni, D. B. 2006. The Nationwide Speech Project: A new corpus of American English dialects. *Speech Communication* 48, 633–644. Clopper, C. G., & Smiljanic, R. 2011. Effects of gender and regional dialect on prosodic patterns in American English. *Journal of Phonetics* 39, 237–245. Clopper, C. G., & Smiljanic, R. 2015. Regional variation in temporal organization in American English. *Journal of Phonetics* 49, 1–15. Coats, S. 2019. A Corpus of regional American language from YouTube. In C. Navarretta et al. (eds.), *Proceedings of the 4th Digital Humanities in the Nordic Countries Conference, Copenhagen, Denmark, March 6–8, 2019*. Aachen, Germany: CEUR, 79–91. De Jong, N.H., & Wempe, T. 2009. Praat script to detect syllable nuclei and measure speech rate automatically. *Behavior research methods* 41(2), 385–390. Getis, A., & Ord, J. K. 1992. The Analysis of Spatial Association by Use of Distance Statistics. *Geographical Analysis* 24(7), 189–206. Giles, H., & Powesland, P. 1975. *Speech Style and Social Evaluation*. London/New York: Academic Press. Goldman-Eisler, F. 1961. The significance of changes in the rate of articulation. *Language and Speech* 4(4), 171–174. Google. 2009. [Automatic captions in YouTube](https://googleblog.blogspot.com/2009/11/automatic-captions-in-youtube.html). Grieve, J. 2016. *Regional variation in written American English*. Cambridge, UK: Cambridge University Press. Jacewicz, E., Fox, R. A., O'Neill, C., & Salmons, J. 2009. Articulation rate across dialect, age, and gender. *Language Variation and Change* 21, 233–256 Jacewicz, E., Fox, R. A., & Wei, L. 2010. Between-speaker and within-speaker variation in speech tempo of American English. *Journal of the Acoustical Society of America* 128(2): 839–50. Kendall, T. 2013. *Speech rate, pause, and sociolinguistic variation: Studies in corpus sociophonetics*. London: Palgrave-Macmillan. ]] --- ### References II .verysmall[ .hangingindent[ Miller, N., Maruyama, G., Beaber, R. J., & Valone, K. 1976. Speed of speech and persuasion. *Journal of Personality and Social Psychology* 34, 615–25. Oller, D. K. 1973. The effect of position in utterance on speech segment duration in English. *Journal of the Acoustical Society of America* 54, 1235–1247. Ord, J. K., & Getis, A. 1995. Local spatial autocorrelation statistics: Distributional issues and application. *Geographical Analysis* 27(4), 286–306. Preston, D. 1989. *Perceptual dialectology: Nonlinguists' views of areal linguistics*. Dordrecht: Foris. Preston, D. 1999. A language attitude approach to the perception of regional variation. In D. Preston (ed.), *The handbook of perceptual dialectology, vol. 1*. Amsterdam: John Benjamins, 359–73. Ray, G., & Zahn, C. 1990. Regional speech rates in the United States: a preliminary analysis. *Communication Research Reports* 7, 34–7. Ray, G., & Zahn, C. 1999. Language attitudes and speech behavior: New Zealand English and Standard American English. *Journal of Language and Social Psychology* 18(3), 310–319. Roach, P. 1998. Myth 18: Some languages are spoken more quickly than others. In L. Bauer & P. Trudgill (eds.), *Language myths*. London/New York: Penguin, 150–158. Smith, B. L., Brown, B., Strong, W. J., & Rencher, A. C. 1975. Effects of speech rate on personality perception. *Language and Speech* 18(2), 145–52. Street, R. L., Jr., & Brady, R. M. 1982. Speech rate acceptance ranges as a function of evaluative domain, listener speech rate, and communication context. *Communication Monographs* 49(4), 290–308. Street, R. L., Jr., Brady, R. M., & Putman, W. B. 1983. The influence of speech rate stereotypes and rate similarity on listeners' evaluations of speakers. *Journal of Language and Social Psychology* 2(1), 37–56. Thakerar, J. N., & Giles, H. 1981. They are – so they speak: Noncontent speech stereotypes. *Language and Communication* 1, 251–256. Tsao, Y.-C., & Weismer, G. 1997. Interspeaker variation in habitual speaking rate: Evidence for a neuromuscular component. *Journal of Speech, Language, and Hearing Research* 40, 858–866. Tsao, Y.-C., Weismer, G., & Iqbal, K. 2006. Interspeaker variation in habitual speaking rate: Additional evidence. *Journal of Speech, Language, and Hearing Research* 49, 1156–1164. United States Census Bureau. 2017. Subcounty Resident Population Estimates: April 1, 2010 to July 1, 2017. [Data set]. https://www2.census.gov/programs-surveys/popest/datasets/2010-2017/cities/totals/sub-est2017_all.csv Yuan, J., Cieri, C., & Liberman, M. 2006. Towards an integrated understanding of speaking rate in conversation. *Proceedings of Interspeech 2006, Pittsburgh, PA*, 541–544. ]]