class: inverse, center, middle background-image: url(https://cc.oulu.fi/~scoats/oululogoRedTransparent.png); background-repeat: no-repeat; background-size: 80px 57px; background-position:right top; exclude: true --- class: title-slide, center <br> ## A Corpus of Regional American Language from YouTube ### Steven Coats <br><br><br> English Philology, University of Oulu, Finland<br> <a href="mailto:steven.coats@oulu.fi">steven.coats@oulu.fi</a><br> 4th DHN Conference, Copenhagen<br> March 8th, 2019<br> .footnote[.verysmall[[<br><br>                                                                                image source](https://i.dailymail.co.uk/i/pix/2012/07/31/article-2180947-144C31A7000005DC-200_634x618.jpg)]] --- layout: true <div class="my-header"><img border="0" alt="W3Schools" src="https://cc.oulu.fi/~scoats/oululogonewEng.png" width="80" height="80"></div> <div class="my-footer"><span>Steven Coats             A Corpus of Regional American Language from YouTube | DHN 2019</span></div> --- <div class="my-header"><img border="0" alt="W3Schools" src="https://cc.oulu.fi/~scoats/oululogonewEng.png" width="80" height="80"></div> <div class="my-footer"><span>Steven Coats             A Corpus of Regional American Language from YouTube | DHN 2019</span></div> ## Outline 1. Data in dialectology: Linguistic atlases and dialect corpora 2. Data collection from YouTube and corpus creation 3. Preliminary analysis: Getis-Ord G<span class='supsub'><sup>*</sup><sub>i</sub></span> statistic, lexical and grammatical variables .footnote[Slides for the presentation are on my homepage at https://cc.oulu.fi/~scoats] --- <div class="my-header"><img border="0" alt="W3Schools" src="https://cc.oulu.fi/~scoats/NewLogoRussianPNG1.png" width="80" height="80"></div> <div class="my-footer"><span>Steven Coats             A Corpus of Regional American Language from YouTube | DHN 2019</span></div> ### American dialectology data .small[ **Data from traditional language atlases** .pull-left[ - Artificial speech situation (questionnaire) - One speaker/one variant per location ➜ implied categoricity .small[(Kretzschmar 2009, Nerbonne 2009, Szmrecsanyi 2011)] - Linguistic Atlas of the Middle and South Atlantic States: "What word is used for a child born out of wedlock?" **Dialect corpora** - Multiple speakers, relative frequencies ➜ more nuanced view of geographical distribution of linguistic forms - UK: Freiburg English Dialects Corpus .small[(transcribed interviews, Szmrecsanyi and Hernández 2007)] - US: Letters to the editor corpus .small[(written texts, Grieve 2016)] - No corpora of transcribed American **speech** with broad geographic coverage] .pull-right[ 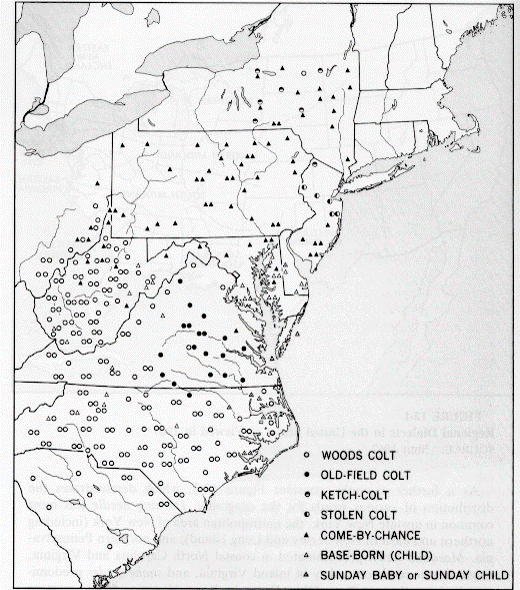 .small[(Kurath 1949)]]] --- ### YouTube automatic speech-to-text captions Billions of YouTube videos, many with speech relevant for dialectological research First automatically generated speech-to-text captions 2009 .small[(Google 2009)] Recent advances in neural-network-based speech-to-text transcription increase transcript accuracy .small[(Chiu et al. 2018)] -- #### Focus on US local government/civic organization meetings Public meetings of elected representatives at town/city/state level: advantages in terms of representativeness and comparability - Speaker place of residence - Similar in terms of topical content and communicative contexts --- ### Example video <iframe width="560" height="315" src="https://www.youtube.com/embed/WY9RPeXA3pw?rel=0&&showinfo=0&cc_load_policy=1&cc_lang_pref=en" frameborder="0" allow="accelerometer; autoplay; encrypted-media; gyroscope; picture-in-picture" allowfullscreen></iframe> --- ### .vtt file 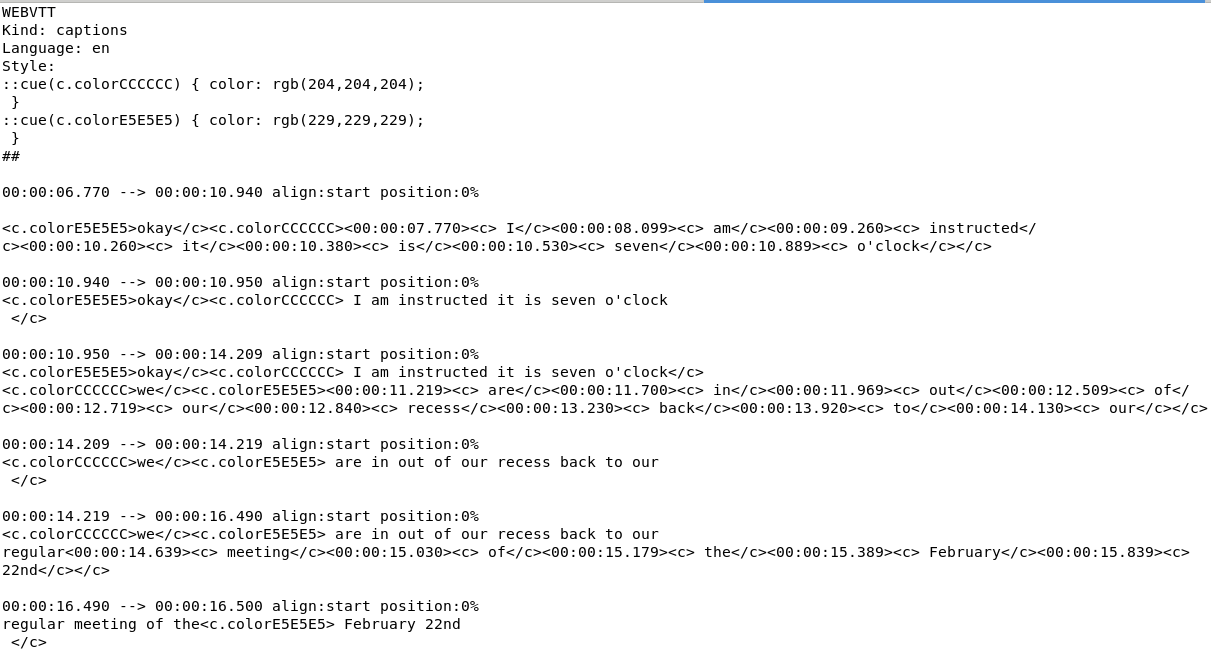 --- ### Data collection Script to search YouTube API for channels: - Substrings `county of`, `city of`, `municipal`, `town meeting`, `city council`, `county supervisors`, `board of supervisors`, `government`, and `official government` + names/abbreviations 50 U.S. states or names of the 312 municipalities and 100 counties by population in the United States + corresponding state names/abbreviation - E.g. `county of Alabama`, `city council CA`, `official government Chicago, Illinois`, `official government Los Angeles County, California` -- #### Data filtering - 1,680 channel matches, remove false positives and duplicates - Geocoding API to assign exact latitude-longitude coordinates .small[(Esmukov et al. 2018)] - 53,743 captions files downloaded in .vtt format, script to extract text and timings - PoS tagging with NLTK .small[(Bird, Loper & Klein 2009)] --- ### Spoken American YouTube Corpus (SpAmYT) - First corpus of spoken language for the entire US with fine geographic granularity - Largest spoken language corpus - 579 locations - 29,267.14 hours of video - 252,259,141 words --- ### Channels sampled .verysmall[(channels with at least 1,000 words)] .verysmall[
] --- ### Exploratory analysis - Channel locations ➜ Voronoi tessalation; relative frequencies ➜ spatial autocorrelation 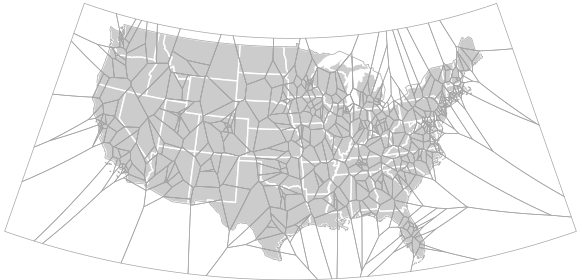 - Proof of concept: How are lexical items pertaining to weather (**snow**, **sun**) spatially distributed? - Copula contraction: Where do Americans use more contracted forms (e.g. **he's**, **they're**) compared to uncontracted forms (e.g. **he is**, **they are**)? --- ### <font>Getis-Ord G<span class='supsub'><sup>*</sup><sub>i</sub></span></font> <font size='3.5'>(Ord & Getis 1992; Getis & Ord 1995)</font> Spatial autocorrelation statistic used in geography and recently in dialectology .small[(e.g. Grieve 2016)] For each point in spatially distributed data: Positive value ➜ in a cluster of high values, negative value ➜ in a cluster of low values, zero ➜ not in a cluster -- `$$G_i^* = \frac{\sum_{j=1}^{n} w_{ij}x_j- \bar{X}\sum_{j=1}^{n} w_{ij}}{s\sqrt{\frac{n\sum_{j=1}^{n} w_{ij}^2 -{(\sum_{j=1}^{n} w_{ij})^2}}{n-1}}}$$` .small[ `\(n\)` = number of locations, `\(i,j\)` = location indexes, `\(x\)` = value of variable, `\(w_{ij}\)` = spatial weight between locations `\(i\)` and `\(j\)`, `\(\bar{X}\)` = mean of `\(x\)`, `\(s\)` = standard deviation of `\(x\)`] Result is a standard deviate (significant at `\(p = 0.05\)` for `\(G_i^*\geq\pm1.645\)`) -- .small[ **Spatial weights** can be binary, based on polygon contiguity, a cutoff distance, or a nearest-neighbor function; or continuous, based on inverse distance or other functions] --- <div class="my-header"><img border="0" alt="W3Schools" src="https://cc.oulu.fi/~scoats/NewLogoRussianPNG1.png" width="80" height="80"></div> <div class="my-footer"><span>Steven Coats             A Corpus of Regional American Language from YouTube | DHN 2019</span></div> ### Exploratory analysis: weather terms <div class="midcenter"> <iframe src="https://cc.oulu.fi/~scoats/DHN19_weather_map.html" style="max-width = 100%" sandbox="allow-same-origin allow-scripts" width="860px" height="500px" scrolling="no" seamless="seamless" frameborder="0" align="top"> </iframe> .vsup[(Weights matrices: 50 nearest-neighbor binary and inverse distance with cutoffs of 200km and 100km)] </div> --- ### Exploratory analysis: copula contraction <div class="midcenter"> <iframe src="https://cc.oulu.fi/~scoats/DHN19_contr_map.html" style="max-width = 100%" sandbox="allow-same-origin allow-scripts" width="860px" height="500px" scrolling="no" seamless="seamless" frameborder="0" align="top"> </iframe> .vsup[(Weights matrices: 50 nearest-neighbor binary)] </div> --- ### Copula contraction: Grieve 2016 .small[(p. 151)] 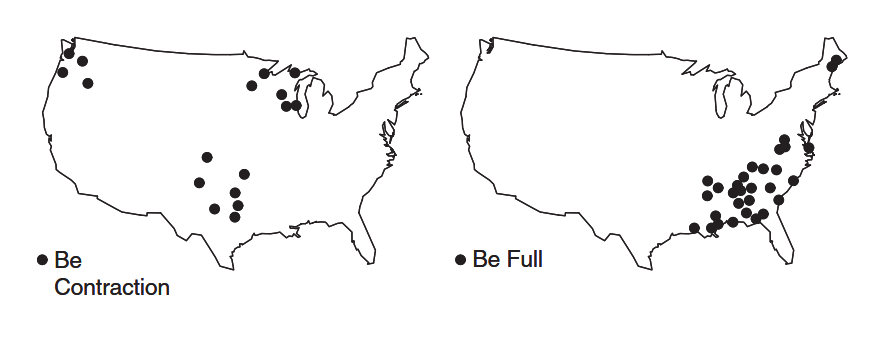 - More contraction in the West, less in the East/Southeast - Spoken-language pattern corresponds to written-language pattern --- ### Summary and outlook - First dialect corpus of spoken American English - First large corpus from automatic speech-to-text transcripts - Largest dialect corpus (252m words), extensive geographical coverage - First corpus from local government/civic organization meetings -- Spatial autocorrelation/visualization shows that - Lexical types show interpretable regional variation - Geographical distribution of copula contraction: spoken language similar to written language -- *** - Analysis of lexico-grammatical features for aggregate dialectometry (cf. Grieve 2016) - NLP-based analyses (regional topics: topic modeling, regional word semantics: word vectors) - Speech/articulation rate analysis (in progress!) - Automatic annotation of speaker variables? --- #Thank you! --- ### References .small[ .hangingindent[ Bird, S., Loper, E. and Klein, E. 2009. *Natural Language Processing with Python*. Newton, MA: O'Reilly. Chiu, C.-C., Sainath, T., Wu, Y., Prabhavalkar, R., Nguyen, P., Chen, Z., Kannan, A., Weiss, R. J., Rao, K., Gonina, E., Jaitly, N., Li, B., Chorowski, J., & Bacchiani, M. 2018. State-of-the-art speech recognition with sequence-to-sequence models. [arXiv:1712.01769v6 [cs.CL]](https://arxiv.org/pdf/1712.01769.pdf). Esmukov, K., et al. 2018. [GeoPy](https://github.com/geopy/geopy) (Python library). Getis, A., & Ord, J. K. 1992. The Analysis of Spatial Association by Use of Distance Statistics. *Geographical Analysis* 24(7), 189–206. Google. 2009. [Automatic captions in YouTube](https://googleblog.blogspot.com/2009/11/automatic-captions-in-youtube.html). Grieve, J. 2016. *Regional variation in written American English*. Cambridge, UK: Cambridge University Press. Kretzschmar, W. A. 2009. *The linguistics of speech*. Cambridge, UK: Cambridge University Pres. Kurath, H. 1949. *A word geography of the Eastern United States*. Ann Arbor, MI: University of Michigan Press. Nerbonne, J. 2009. Data-driven dialectology. *Language and Linguistics Compass* 3(1), 175–198. Ord, J. K. & Getis, A. 1995. Local spatial autocorrelation statistics: Distributional issues and application. *Geographical Analysis* 27(4), 286–306. Szmrecsanyi, B. 2011. Corpus-based dialectometry: a methodological sketch. *Corpora* 6(1), 45–76. Szmrecsanyi, B. & Hernández, N. 2007. [Manual of information to accompany the Freiburg Corpus of English Dialects Sampler (FRED-S)](https://freidok.uni-freiburg.de/data/2859). Freiburg: University of Freiburg. ]]