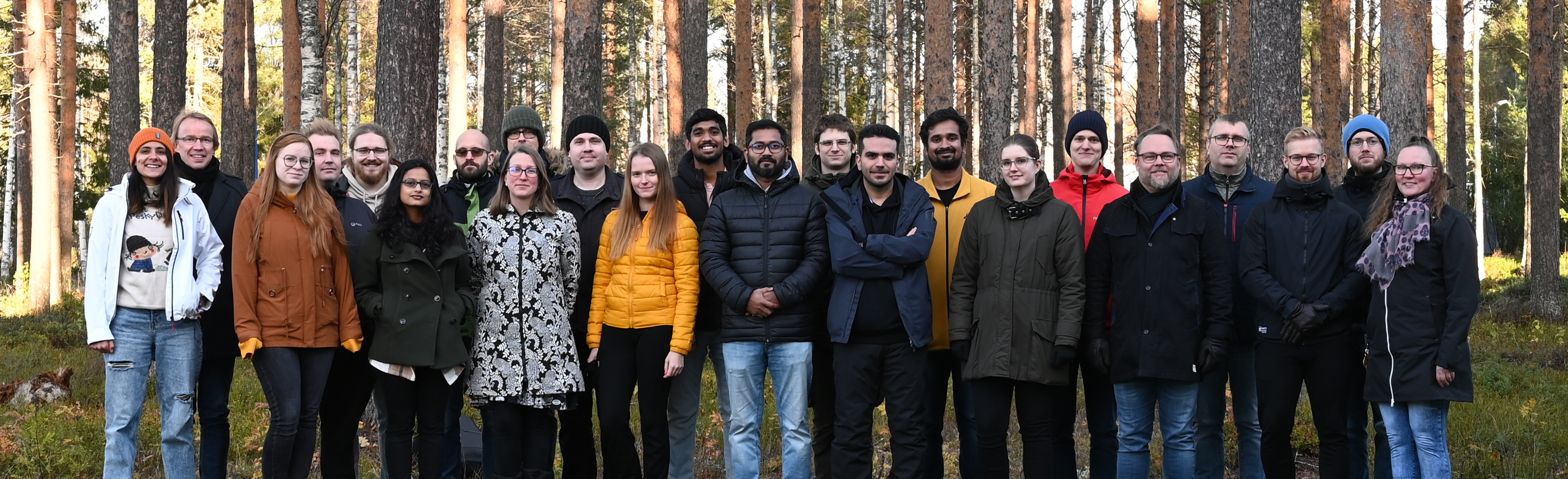
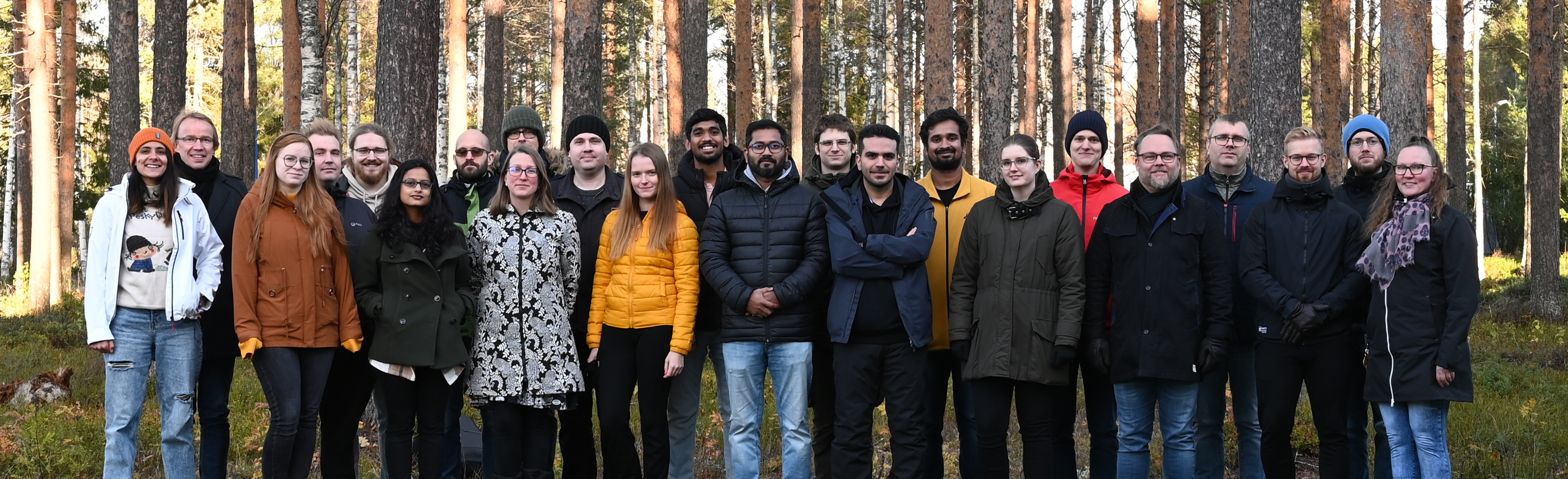
office: KE 318
email: firstname.lastname@oulu.fi
ORCID: 0000-0002-7793-3306
ResearchGate: Ouail Zakary
My research focuses on developing advanced machine learning-driven approaches for modeling large-scale molecular systems under real physicochemical conditions. By integrating state-of-the-art quantum mechanics at the relativistic level and molecular dynamics simulations with atomistic machine learning, I aim to deliver highly predictive models for both structural and dynamic processes.
A significant part of my work involves training graph neural networks, along with Kernel and Gaussian Process regression models, to develop machine learning interatomic potentials and machine learning-based NMR parameter models. These models enable long-timescale simulations, improving our ability to analyze experimental NMR data and predict new experimental outcomes for complex materials.